- Gonzaga
- Norfolk State
- Oklahoma
- Missouri
- Creighton
- UCSB
- Virginia
- Ohio
- USC
- Drake
- Kansas
- Eastern Washington
- Oregon
- VCU
- Iowa
- Grand Canyon
- Michigan
- Texas Southern
- LSU
- St. Bonaventure
- Colorado
- Georgetown
- Florida State
- UNC Greensboro
- BYU
- UCLA
- Texas
- Abilene Christian
- UConn
- Maryland
- Alabama
- Iona
- Gonzaga
- Oklahoma
- Creighton
- Virginia
- USC
- Kansas
- Oregon
- Iowa
- Michigan
- St. Bonaventure
- Colorado
- Florida State
- BYU
- Texas
- UConn
- Alabama
- Gonzaga
- Virginia
- USC
- Iowa
- Michigan
- Florida State
- Texas
- Alabama
- Gonzaga
- Iowa
- Baylor
- Ohio State
- Michigan
- Alabama
- Illinois
- Houston
- Iowa
- Michigan
- Ohio State
- Illinois
- Michigan
- Illinois
- Baylor
- Villanova
- Arkansas
- Ohio State
- Illinois
- Tennessee
- San Diego
- Houston
- Baylor
- Wisconsin
- Villanova
- Purdue
- Texas Tech
- Arkansas
- Virginia Tech
- Ohio State
- Illionois
- Loyola
- Tennessee
- Oklahoma
- San Diego
- West Virginia
- Rutgers
- Houston
- Baylor
- Hartford
- North Carolina
- Wisconsin
- Villanova
- Winthrop
- Purdue
- North Texas
- Texas Tech
- Utah State
- Arkansas
- Colgate
- Florida
- Virginia Tech
- Ohio State
- Oral Roberts
- Illinois
- Drexel
- Loyola
- Georgia Tech
- Tennessee
- Oregon State
- Oklahoma State
- Liberty
- San Diego State
- Syracuse
- West Virginia
- Morehead
- Clemson
- Rutgers
- Houston
- Cleveland State
- Gonzaga
- Norfolk State
- Oklahoma
- Missouri
- Creighton
- UCSB
- Virginia
- Ohio
- USC
- Drake
- Kansas
- Eastern Washington
- Oregon
- VCU
- Iowa
- Grand Canyon
- Michigan
- Texas Southern
- LSU
- St. Bonaventure
- Colorado
- Georgetown
- Florida State
- UNC Greensboro
- BYU
- UCLA
- Texas
- Abilene Christian
- UConn
- Maryland
- Alabama
- Iona
- Gonzaga
- Oklahoma
- Creighton
- Virginia
- Drake
- Kansas
- VCU
- Iowa
- Michigan
- St. Bonaventure
- Georgetown
- Florida State
- BYU
- Texas
- UConn
- Alabama
- Gonzaga
- Virginia
- USC
- Iowa
- Michigan
- Georgetown
- BYU
- UConn
- Gonzaga
- Iowa
- North Carolina
- Utah State
- Georgetown
- BYU
- Liberty
- Houston
- Gonzaga
- Georgetown
- North Carolina
- Houston
- Georgetown
- Houston
- North Carolina
- Villanova
- Utah State
- Oral Roberts
- Illinois
- Liberty
- San Diego
- Houston
- Hartford
- North Carolina
- Villanova
- North Texas
- Utah State
- Colgate
- Florida
- Oral Roberts
- Drexel
- Georgia Tech
- Tennessee
- Liberty
- San Diego
- Morehead State
- Rutgers
- Houston
- Baylor
- Hartford
- North Carolina
- Wisconsin
- Villanova
- Winthrop
- Purdue
- North Texas
- Texas Tech
- Utah State
- Arkansas
- Colgate
- Florida
- Virginia Tech
- Ohio State
- Oral Roberts
- Illinois
- Drexel
- Loyola
- Georgia Tech
- Tennessee
- Oregon State
- Oklahoma State
- Liberty
- San Diego State
- Syracuse
- West Virginia
- Morehead
- Clemson
- Rutgers
- Houston
- Cleveland State
- Gonzaga
- Norfolk State
- Oklahoma
- Missouri
- Creighton
- UCSB
- Virginia
- Ohio
- USC
- Drake
- Kansas
- Eastern Washington
- Oregon
- VCU
- Iowa
- Grand Canyon
- Michigan
- Texas Southern
- LSU
- St. Bonaventure
- Colorado
- Georgetown
- Florida State
- UNC Greensboro
- BYU
- UCLA
- Texas
- Abilene Christian
- UConn
- Maryland
- Alabama
- Iona
- Gonzaga
- Oklahoma
- Creighton
- Virginia
- USC
- Kansas
- Oregon
- Iowa
- Michigan
- LSU
- Colorado
- Florida State
- BYU
- Texas
- UConn
- Alabama
- Gonzaga
- Virginia
- Kansas
- Iowa
- Michigan
- Florida State
- Texas
- Alabama
- Gonzaga
- Iowa
- Baylor
- Ohio State
- Michigan
- Alabama
- Illinois
- Houston
- Gonzaga
- Michigan
- Baylor
- Illinois
- Gonzaga
- Illinois
- Baylor
- Purdue
- Arkansas
- Ohio State
- Illinois
- Oklahoma State
- West Virginia
- Houston
- Baylor
- North Carolina
- Villanova
- Purdue
- Texas Tech
- Arkansas
- Florida
- Ohio State
- Illionois
- Loyola
- Tennessee
- Oklahoma State
- San Diego
- West Virginia
- Clemson
- Houston
- Baylor
- Hartford
- North Carolina
- Wisconsin
- Villanova
- Winthrop
- Purdue
- North Texas
- Texas Tech
- Utah State
- Arkansas
- Colgate
- Florida
- Virginia Tech
- Ohio State
- Oral Roberts
- Illinois
- Drexel
- Loyola
- Georgia Tech
- Tennessee
- Oregon State
- Oklahoma State
- Liberty
- San Diego State
- Syracuse
- West Virginia
- Morehead
- Clemson
- Rutgers
- Houston
- Cleveland State
Motivation and Background
Russian Literature & French Statistics
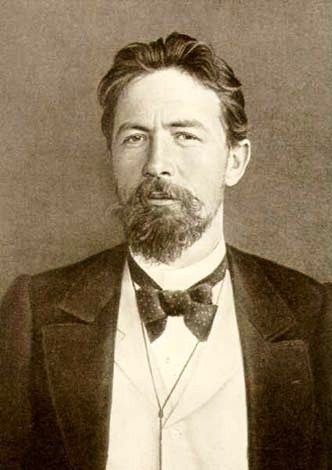
In Anton Chekhov’s 1894 story, “The Student”, Ivan is heading home during a cold March evening. He just left from an encounter with Vasilisa, who cried bitterly when he related to her the Biblical story about Peter’s betrayal that was described as occuring 2,000 years ago.
He realizes that it wasn’t the way he told the story that moved her, but rather the guilt that Peter himself felt that brought this emotion from Vasilisa.
Ivan then says to himself,
” ‘the past […] is linked with the present by an unbroken chain of events flowing one out of another’ “
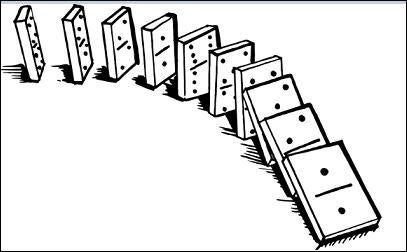
“[…] it seemed to him that he had just seen both ends of that chain; that when he touched one end the other quivered.”
This chain of causality that Chekhov described was not a new idea in the late 19th century. Earlier in 1814, the French polymath, Pierre-Simon Laplace, wrote in his book A Philosophical Essay on Probabilities
“Present events are connected with preceding ones by a tie based upon the evident principle that a thing cannot occur without a cause which produces it.”
He then proposed a thought: if a sufficiently intelligent being knew the present state of every single granularity of the Universe, that is, every causal link, then this being would be able to perfectly predict the future as well as retrace the past.
“[…] an intelligence which could comprehend all the forces by which nature is animated and the respective situation of the beings who could compose it - an intelligence sufficiently vast to submit these data to analysis […] for it; nothing would be uncertain and the future, as the past, would be present to its eyes”
As if an approximation to this hypothetical intelligence, the field of computational statistical learning emerged as a way to predict outcome using historical data. Writing about the positive examples of these algorithms would further indulge a field already saturated with promises of the future.
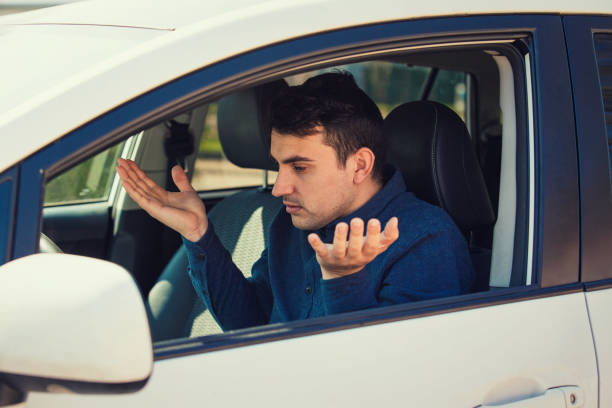
And this field doesn’t gate-keep either.
With publicly available packages such as sklearn, keras, and tensorflow the bar to start using sophisticated algorithms has never been lower.
In conjunction with the availability of open-source datasets, it seems that every field is now open to modeling.
This field promises: not everyone can be an expert, but with the right tools and resources, they can create models that perform like experts.
American Basketball
But can we really explore a field for which we have no “domain knowledge” of and create predictions that suprass the foresight of experts? The term “domain knowledge” refers to the traditional method of becoming acquintated with any particular field: recieving an accredited degree, consulting the knowledge of the past using textbooks, or by simply being an aged observer of the phenomenon.
This question is especially relevant when we deal with human-centered fields.
For example, within the neary 100-year history of the NCAA’s college basketball tournemant “March Madness”, a 16-seeded team has never won against a 1-seeded team. That is, until 2018.
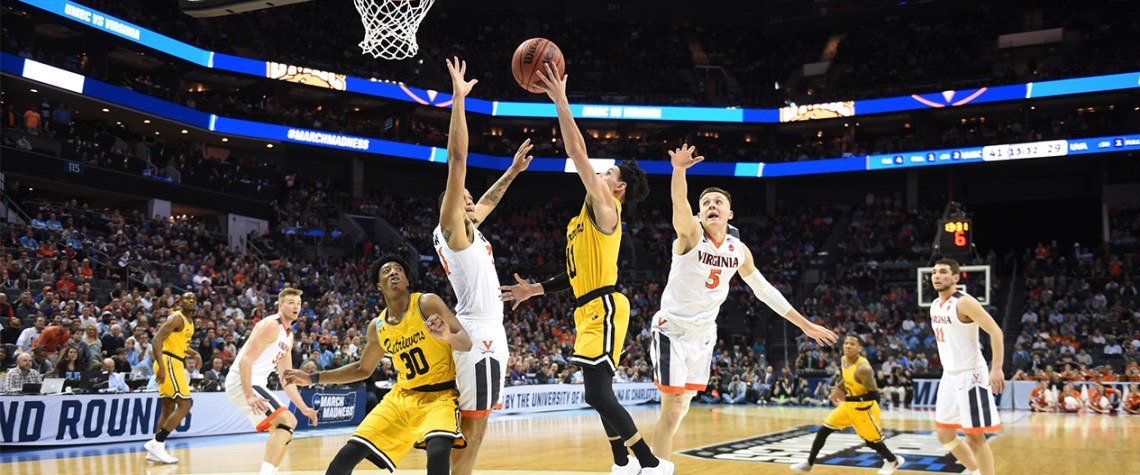
Could an algorithm have predicted this performance, even though no basketball “expert” could?
In 2018 I trained a classifier on team-ranking data from basketball enthusiasts. It gave UMBC a 2% chance of victory. Perhaps a “better” model would have expressed the historical impossibility with greater sufficiency and given it a 0% chance.
This year, my methodology hasn’t changed. Instead, I take a step back and ask: “do I even bother modelling a college basketball when I myself have never casually watched sports?”
So, I compare my work to the following “non-data driven” bracket predictions:
- the winner always has the more popular team color.
- the winner is chosen by the wisdom of crowds
Through this comparison, I begin asking:
- Is it just as bad to guess (or forgo data when making predictions) than create a model for a poorly-understood field?
- Does all data point to an interesting & predictive model?
- Are there fields where algorithms will never be as good as the opinion of “experts”
- Do experts even exist when it comes to “prediction”?